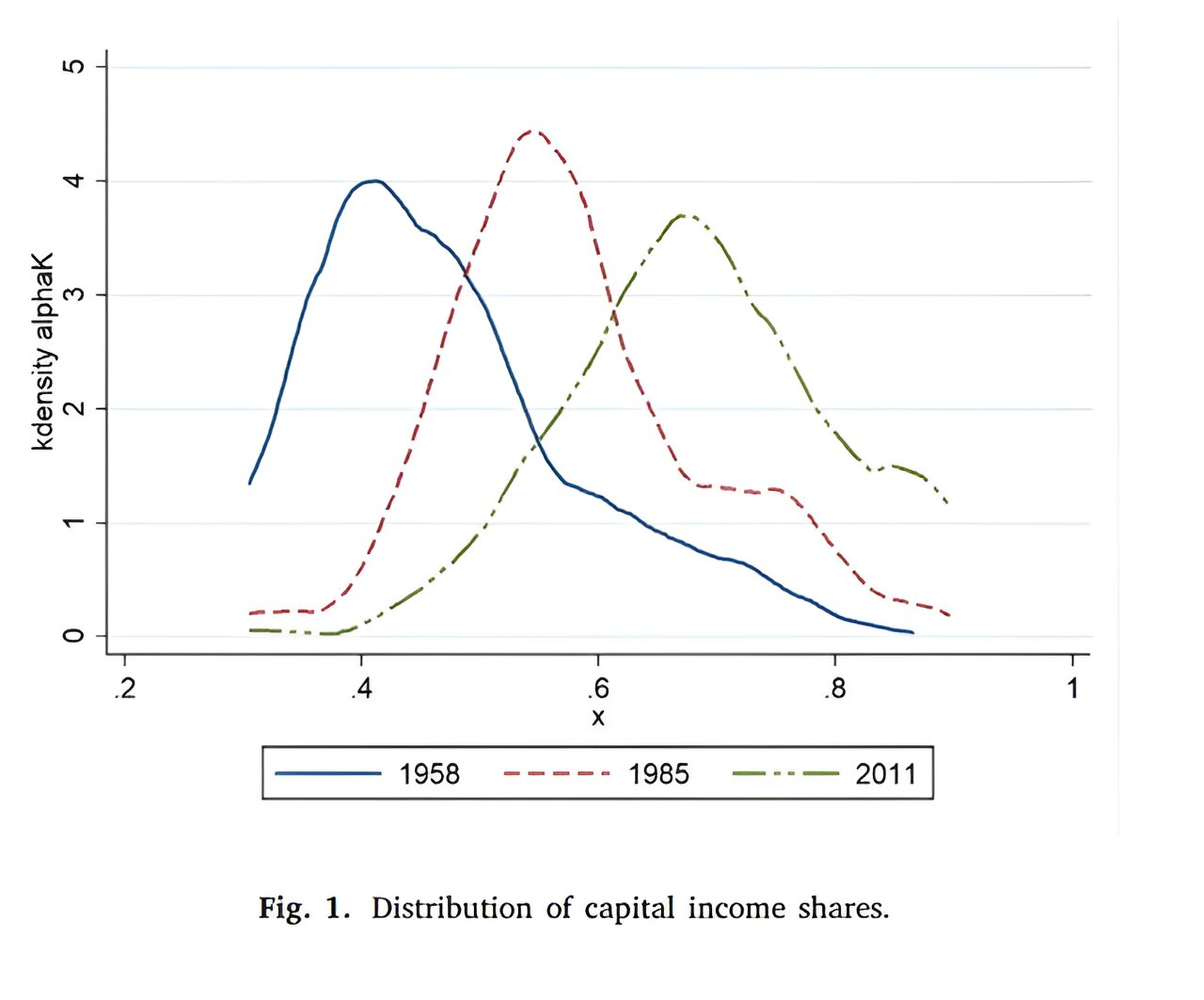
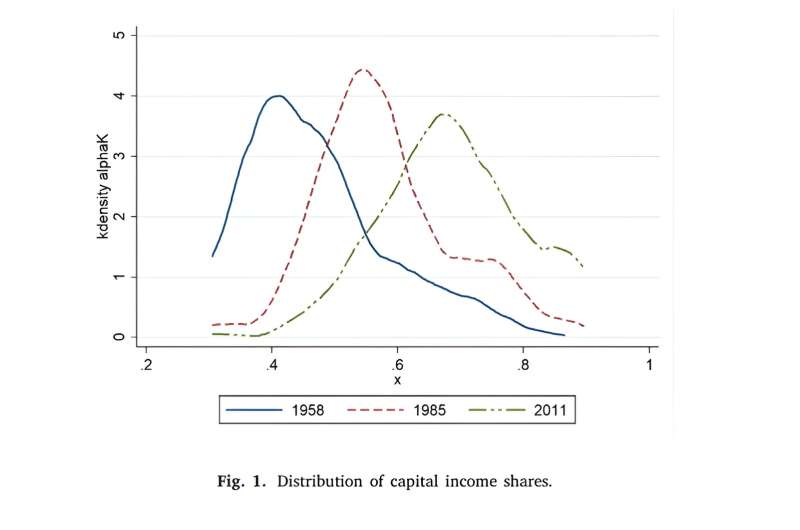
Assistant Professor Liu Zhengwen and Professor Zhang Bo of the School of Economics, together with Professor Justin Yifu Lin from the Institute of New Structural Economics and National School of Development have co-authored an article titled “Endowment, technology choice, and industrial upgrading.” It has been published in Structural Change and Economic Dynamics.
Employing a dynamic infinite-industry model, the paper discusses the impact of factor endowments on technology choice and industrial upgrading.
The industrial sector, which refers to all manufacturing industries, traditionally characterized as “the main engine of fast growth” exhibits two patterns as capital endowment becomes more abundant: first, the production technology in each individual industry becomes more capital-intensive, and second, the more capital-intensive industries gain a larger market share within the industrial sector.
This paper aims to explain these observations by answering the following questions: how does the aggregate endowment structure determine technology choices within each industry and the aggregate industrial structure? Furthermore, how do dynamics in endowment structure, technology choices, and industrial structural interrelate?
The three professors first document the capital intensity and value-added share of each industry in the economy, using the National Bureau of Economic Research—U.S. Census Bureau’s Center for Economic Studies (NBERCES) Manufacturing Industry Database from 1958 to 2011. They find a strong pattern that capital intensity in each industry, as well as in the whole industrial sector, is increasing over time.
Their findings reveal that the distribution of market shares, measured by the value-added share of each industry in the total industrial sector, is unimodal. In addition, they observe that the leading industry, which has the largest market share in the industrial sector, shifts to more capital-intensive industries as the economy becomes more capital abundant. This pattern is persistent using the NBER-CES Manufacturing Industry Database and the China Industrial Productivity (CIP) data set, as well as the United Nations Industrial Development Organization (UNIDO) data set for cross-country analysis.
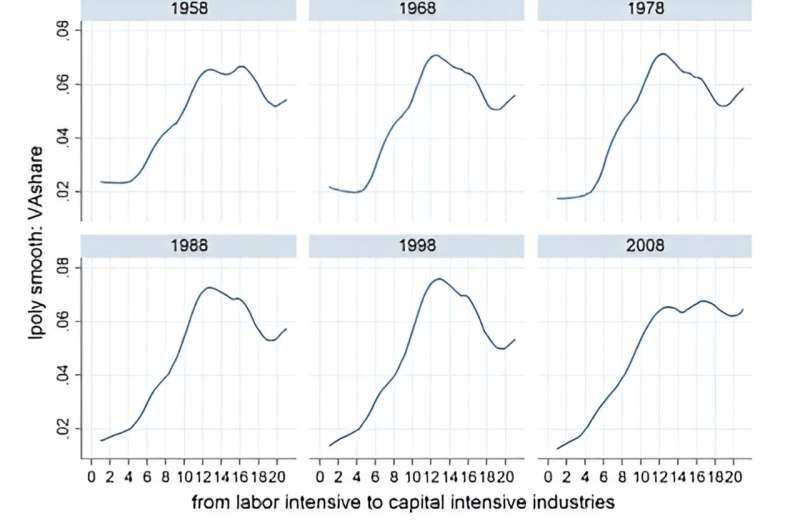
To capture the above empirical facts, the authors set up a dynamic infinite industry general equilibrium model. A remarkable feature of their model is the consideration of technology choice, by which a firm selects a specific technology from a set of available technologies for conducting production within a certain industry. The model produces results that align with the aforementioned stylized facts. In the baseline model, technology choice is costless.
However, in practice, costs may arise due to difficulties in acquiring patents, specific human capital requirements for different technologies, and specific infrastructure investment necessary to facilitate adoption. The professors provide an extension of the model to incorporate the cost of technology choices. In practice, these cost can be too high, leading to the complete cessation of technological progress and industrial upgrading. In such cases, government intervention would be necessary.
In the section of testing stylized fact, Assistant Professor Liu Zhengwen and Professor Zhang Bo and Professor Justin Yifu Lin demonstrate that the capital intensities are heterogeneous across different industries and change over time. They employ the NBER-CES Manufacturing Industry Database, which covers 473 industries at the 6-digit North American Industry Classification System (NAICS) level from 1958 to 2011. Supplementary data from the UNIDO data set are also used, which cover 148 countries and 18 industries from 1963 to 2014.
Subsequently, the professors demonstrate the relationship between market share and average capital intensity across industries, separately for the United States and China, using the NBER-CES Manufacturing Industry Database and the China Industrial Productivity (CIP) data set. Finally, using the UNIDO data set, the authors conduct a panel data regression and show that the pattern they illustrate is universal.
Finally, the authors use the NBER-CES data set from 1958 to 2011 to compute the technology choice and technology frontier of each industry. Employing the estimated parameters, they simulate the model quantitatively and conduct counterfactual analysis.
This paper characterizes the evolution of the industrial sector during economic growth both empirically and theoretically.
The key findings are that, first, technology in each manufacturing industry, as well as the whole industrial sector, becomes more capital-intensive as the economy becomes more capital abundant. Second, the industrial structure is unimodal, with a leading industry at any time in the process of economic development; with the accumulation of capital endowment, the industrial structure shifts more and more to capital-intensive industries, and the leading industry is taken over by a new leading industry continuously.
The model of the paper shows that the factor endowment structure is crucial in determining technology choice and industrial structure. The dynamic model captures the interaction among endowment structure, technological choices, and industrial structure. The technology choices and industrial structure are determined by given endowments in each period. As time goes by, the economy accumulates capital, and the aggregate endowment structure becomes more capital abundant. Notably, the optimal technology choices and industrial structure guarantee the fastest speed in accumulating capital. In this way, their model captures the dynamic process of industrialization.
More information:
Justin Yifu Lin et al, Endowment, technology choice, and industrial upgrading, Structural Change and Economic Dynamics (2023). DOI: 10.1016/j.strueco.2023.03.002
Provided by
Peking University
Citation:
Study discusses impact of factor endowments on technology choice, industrial upgrading (2023, December 15)
retrieved 16 December 2023
from https://phys.org/news/2023-12-discusses-impact-factor-endowments-technology.html
This document is subject to copyright. Apart from any fair dealing for the purpose of private study or research, no
part may be reproduced without the written permission. The content is provided for information purposes only.
download dice dream rewards app free for android ste primo
beach buggy racing for android download the apk from uptodown
The Art of Earning TikTok Coins
مستقبل العملات TikTok: التوجهات والتوقعات
LivU Video Chat Free Coin Generators: Fact or Fiction?
Mastering Free Spins in Coin Master: Expert Insights
Legit Ways to Get Avacoins in Avakin Life for Free
Bingo Blitz Credits Farming Techniques: Unveiled
Coin Master Spin Hack Safety: Best Practices
genshin impact 3 4 codes january 2023 free primogems mora
ZEPETO Zems Hack: A Complete Overview
The Art of Earning TikTok Coins
تحسين تجربتك في TikTok مع العملات المجانية
Unlocking Kirin Money in Free Fire: A Comprehensive Guide
The Future of Free Spins in Coin Master: Trends and Predictions
Avakin Life Avacoins Farming Strategies: Success Tips
Mastering Credits in Bingo Blitz: Expert Insights
How to Get Spins in Coin Master Legally and Quickly
all redeem codes in january 2023 for free primogems and mora
ZEPETO Zems Farming: Tips for Success
match masters free gifts coins and boosters pocket tactics
Free TikTok Coins: The Easiest Methods
Free Gems in Brawl Stars Today: Quick Tips
dragon city mobile apps on google play