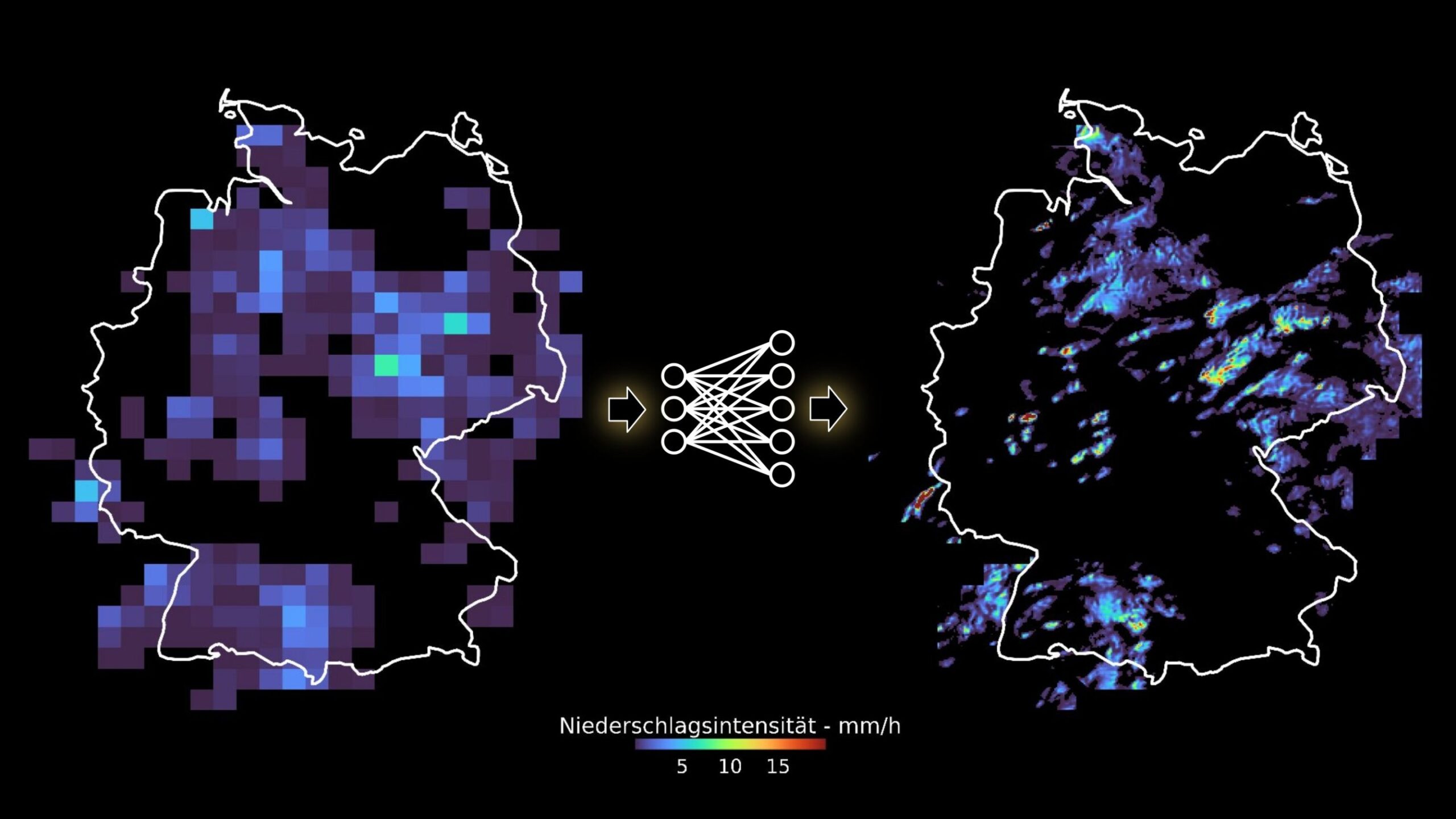
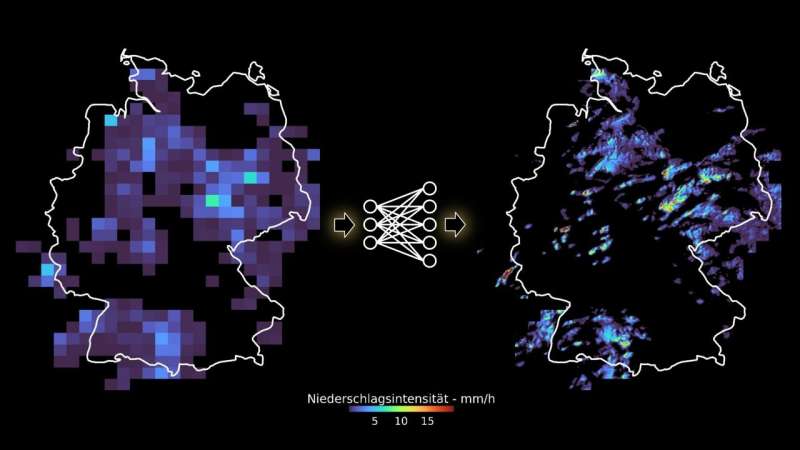
Strong precipitation may cause natural disasters, such as floodings or landslides. Global climate models are required to forecast the frequency of these extreme events, which is expected to change as a result of climate change. Researchers of Karlsruhe Institute of Technology (KIT) have now developed a first method based on artificial intelligence (AI), by means of which the precision of coarse precipitation fields generated by global climate models can be increased.
The researchers succeeded in improving spatial resolution of precipitation fields from 32 to two kilometers and temporal resolution from one hour to 10 minutes. This higher resolution is required to better forecast the more frequent occurrence of heavy local precipitation and the resulting natural disasters in future. The study is published in the journal Earth and Space Science.
Many natural disasters, such as floodings or landslides, are directly caused by extreme precipitation. Researchers expect that increasing average temperatures will cause extreme precipitation events to further increase. To adapt to a changing climate and prepare for disasters at an early stage, precise local and global data on the current and future water cycle are indispensable.
“Precipitation is highly variable in space and time and, hence, difficult to forecast, in particular on the local level,” says Dr. Christian Chwala from the Atmospheric Environmental Research Division of KIT’s Institute of Meteorology and Climate Research (IMK-IFU), KIT’s Campus Alpine in Garmisch-Partenkirchen.” For this reason, we want to enhance the resolution of precipitation fields generated, e.g., by global climate models and improve their classification as regards possible threats, such as floodings.”
Higher resolution for more precise regional climate models
Currently used global climate models are based on a grid that is not fine enough to precisely present the variability of precipitation. Highly resolved precipitation maps can only be produced with computationally expensive and, hence, spatially or temporally limited models.
“For this reason, we have developed an AI-based generative neural network, called GAN, and trained it with high-resolution radar precipitation fields. In this way, the GAN learns how to generate realistic precipitation fields and derive their temporal sequence from coarsely resolved data,” says Luca Glawion from IMK-IFU.
“The network is able to generate highly resolved radar precipitation films from very coarsely resolved maps.” These refined radar maps not only show how rain cells develop and move, but precisely reconstruct local rain statistics and the corresponding extreme value distribution.
“Our method serves as a basis to increase the resolution of coarsely grained precipitation fields, such that the high spatial and temporal variability of precipitation can be reproduced adequately and local effects can be studied,” says Julius Polz from IMK-IFU.
“Our deep learning method is quicker by several orders of magnitude than the calculation of such highly resolved precipitation fields with numerical weather models usually applied to regionally refine data of global climate models.”
The researchers point out that their method also generates an ensemble of different potential precipitation fields. This is important, as a multitude of physically plausible highly resolved solutions exists for each coarsely resolved precipitation field. Similar to a weather forecast, an ensemble allows for a more precise determination of the associated uncertainty.
Higher resolution for better forecasts under climate change
The results show that the AI model and methodology developed by the researchers will enable future use of neural networks to improve the spatial and temporal resolution of precipitation calculated by climate models. This will allow for a more precise analysis of the impacts and developments of precipitation in a changing climate.
“In a next step, we will apply the method to global climate simulations that transfer specific large-scale weather situations to a future world with a changed climate, e.g., to the year of 2100. The higher resolution of precipitation events simulated with our method will allow for a better estimation of the impacts the weather conditions that caused the flooding of the river Ahr in 2021 would have had in a world warmer by 2 degrees,” Glawion explains. Such information is of decisive importance to develop climate adaptation methods.
More information:
Luca Glawion et al, spateGAN: Spatio‐Temporal Downscaling of Rainfall Fields Using a cGAN Approach, Earth and Space Science (2023). DOI: 10.1029/2023EA002906
Provided by
Karlsruhe Institute of Technology
Citation:
Researchers use deep learning to enhance spatial, temporal resolution of coarse precipitation maps (2023, December 13)
retrieved 13 December 2023
from https://phys.org/news/2023-12-deep-spatial-temporal-resolution-coarse.html
This document is subject to copyright. Apart from any fair dealing for the purpose of private study or research, no
part may be reproduced without the written permission. The content is provided for information purposes only.