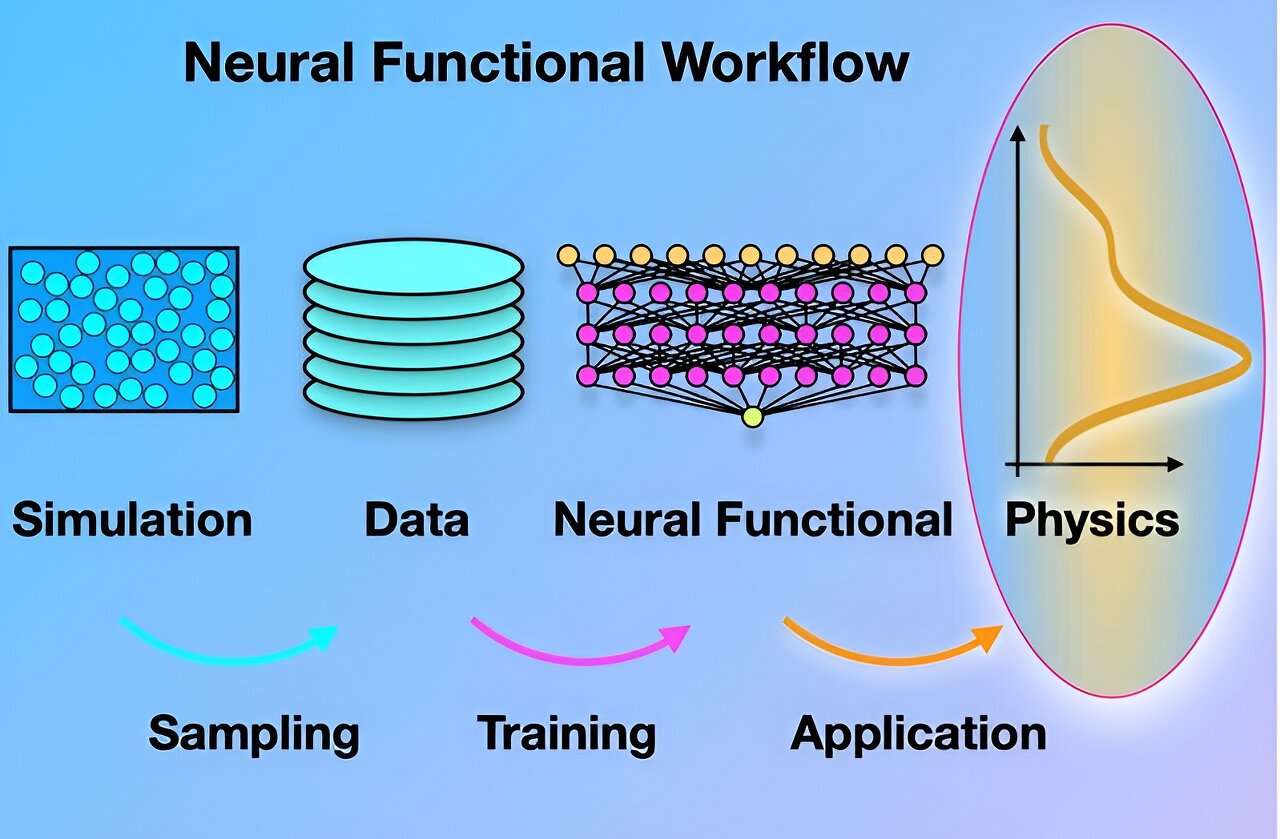
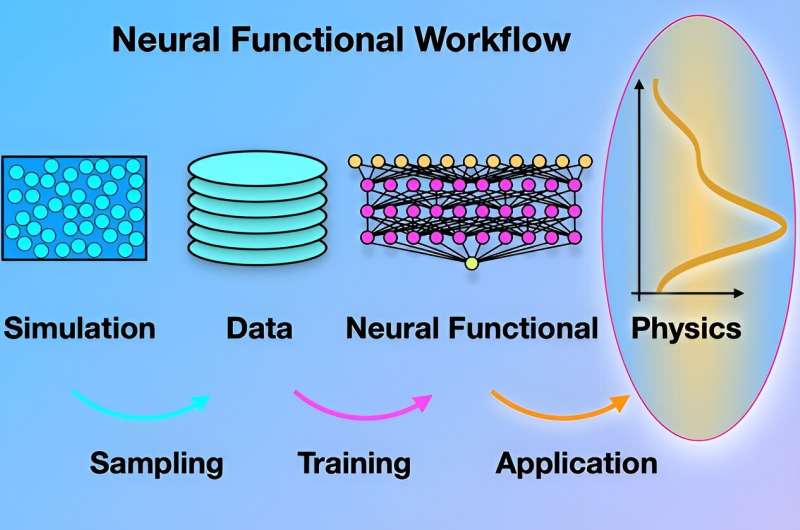
Scientists from Bayreuth have developed a new method for studying liquid and soft matter using artificial intelligence. In a study now published in the Proceedings of the National Academy of Sciences, they open up a new chapter in density functional theory.
We live in a highly technologized world where basic research is the engine of innovation, in a dense and complex web of interrelationships and interdependencies. The published research provides new methods that can have a great influence on widespread simulation techniques, so that complex substances can be investigated on computers more quickly, more precisely and more deeply.
In the future, this could have an influence on product and process design. The fact that the structure of liquids can be excellently represented by the newly formulated neural mathematical relationships is a major breakthrough that opens up a range of possibilities for gaining deep physical insights.
“In the study, we demonstrate how artificial intelligence can be used to carry out fundamental theoretical physics that addresses the behavior of fluids and other complex soft matter systems,” says Prof. Dr. Matthias Schmidt, chair of Theoretical Physics II at the University of Bayreuth. “We have developed an advanced scientific method to study matter at the atomic and (macro)molecular level, combining machine learning and mathematical methods to calculate complex physical properties.”
The Bayreuth researchers present a hybrid scheme based on classical density functional theory and machine learning to determine the equilibrium structure and thermodynamics of fluids under a variety of influences. Schmidt says, “We demonstrate the use of the neural functional in the self-consistent computation of density profiles. The quality of the results exceeds the state of the art of fundamental-measure density functional theory. The results establish machine learning of functionals as an efficient tool for the multiscale description of soft matter.”
Thus, fundamental insights into the structure of matter are gained. The type of matter can be mundane, but it can also be the basis of technological processes and commercial products. “This powerful combination of essentially simple basic techniques has opened a new chapter in density functional theory,” says Schmidt, “because networks trained by simulation data are more accurate than the currently best theoretical approximations designed ‘by hand,’ i.e., with paper and pencil.
“In addition to the significance for the particular field of statistical mechanics of soft matter, I think our method also raises fundamental questions about the human self-understanding of our intellectual activity. For myself, our study gives considerable hope for developments where artificial intelligence, rather than replacing us, expands us in a way that I find very surprising.”
The researchers at the University of Bayreuth also provide broadly accessible tutorial material to accompany the PNAS publication. This includes a further introductory article posted to the arXiv preprint server (“Why neural functionals suit statistical mechanics,” by Florian Sammüller, Sophie Hermann and Matthias Schmidt) as well as programming code available online, which interested people can try out for themselves and work with.
More information:
Florian Sammüller et al, Neural functional theory for inhomogeneous fluids: Fundamentals and applications, Proceedings of the National Academy of Sciences (2023). DOI: 10.1073/pnas.2312484120
Florian Sammüller et al, Why neural functionals suit statistical mechanics, arXiv (2023). DOI: 10.48550/arxiv.2312.04681
Provided by
Bayreuth University
Citation:
AI method for describing soft matter opens up new chapter in density functional theory (2023, December 13)
retrieved 13 December 2023
from https://phys.org/news/2023-12-ai-method-soft-chapter-density.html
This document is subject to copyright. Apart from any fair dealing for the purpose of private study or research, no
part may be reproduced without the written permission. The content is provided for information purposes only.